Samantha Williams (University of York, UK)
Club de Machine Learning
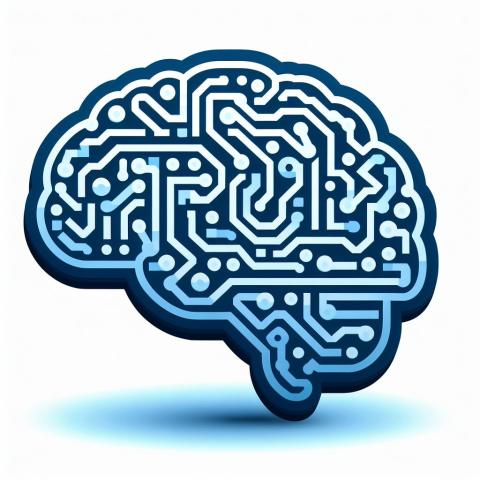
Multivariate kernel density modelling of phonemes for L1 identification
Samantha Williams, University of York, UK
The identification of a speaker’s L1 from their L2 speech is a challenging task. Differences in factors such as proficiency and regional variations can create inconsistencies within an L1 group, and similar target pronunciations mean differences between L1s can be subtle. In forensic applications, additional requirements for transparency in analysis necessitate the use of interpretable methods. This talk will focus on the Multivariate Kernel Density (MVKD) methodology used in a recent language identification study to model vowel phonemes from L2 English speakers. The talk will highlight the insights gained from MVKD in understanding the classification rates and common misidentifications, along with next steps towards working with higher-dimensional data.
Samantha Williams is a PhD candidate in Forensic Speech Science at the University of York, holding a master’s degree in the same field. She received her bachelor’s degree in Electrical and Biomedical Engineering from McMaster University in Canada, where she was involved in research into rTMS and Human-Computer Interface technologies employing EEG and motion tracking. She is interested in the intersection of linguistics, engineering, and technology. Her current research focuses include L1 recognition from L2 speech, interpretable machine learning, and sociophonetics.
Github du club : https://github.com/pmouches/CRNL_ML_Meetings
CRNL | CH Le Vinatier | Bâtiment 462 Neurocampus Michel Jouvet | Room F28 | 95 Boulevard Pinel | 69500 Bron