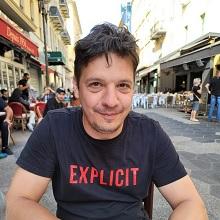
Dezső NEMETH (MEMO CRNL) published a paper in PLoS Computational Biology in collaboration with Peter DAYAN and Noémi ÉLTETO of Max Planck Institute for Biological Cybernetics, Tubingen.
“Our skills become much faster with practice because our brain learns the relationships among all the steps of a skill and can anticipate an upcoming step. In this paper, the authors used a Bayesian sequence model, based on a hierarchy of chunks, to track the participants' skill formation online. The model seamlessly combines predictive information from shorter and longer chunks, weighing them proportionally to their predictive power. Unlike many other seq models, this model is transparent! It not only makes trial-by-trial predictions for participants' speed but also indicates how and how many previous elements influenced individual participants’ internal predictions and how this changed with practice.“
Éltető N, Nemeth D, Janacsek K, Dayan P (2022) Tracking human skill learning with a hierarchical Bayesian sequence model. PLOS Computational Biology 18(11): e1009866. https://doi.org/10.1371/journal.pcbi.1009866